Statistics Seminar (2019-16)
Title: On Rank Estimators in Increasing Dimensions
Speaker: Yanqin Fan, University of Washington
Time: Tuesday, June 11, 14:00-15:00
Place: Room 216, Guanghua Building 2
Abstract:
The family of rank estimators, including Han's maximum rank correlation estimator (Han, 1987) as a notable example, has been widely exploited in studying regression problems. For these estimators, although the linear index is introduced for alleviating the impact of dimensionality, the effect of large dimension on inference is rarely studied. This paper fills this gap via studying the statistical properties of a larger family of M-estimators, whose objective functions are formulated as U-processes and may be discontinuous in increasing dimension set-up where the number of parameters, pn, in the model is allowed to increase with the sample size, n. First, we find that often in estimation, as pn=n! 0, (pn=n)1=2 rate of convergence is obtainable. Second, we establish Bahadur-type bounds and study the validity of normal approximation, which we find often requires a much stronger scaling requirement than p2n=n ! 0. Third, we state conditions under which the numerical derivative estimator of asymptotic covariance matrix is consistent, and show that the step size in implementing the covariance estimator has to be adjusted with respect to pn. All theoretical results are further backed up by simulation studies.
Introduction:
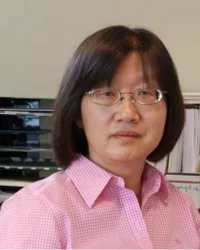
Yanqin Fan is a Castor Professor of Economics at University of Washington. Her recent research focuses on partial identification of distributional treatment effects and uniform inference, inequality-constrained estimation and inference, estimation and inference in increasing dimension, and modal regression. She has published her work in leading journals in economics/econometrics/statistics including Econometrica, Review of Economic Studies, Journal of the American Statistical Association, Journal of Econometrics, and Econometric Theory. She is currently serving as AE for several journals including Journal of Econometrics, Journal of Financial Econometrics, and Econometric Reviews.
Title:Info-Metrics for Modeling and Inference
Speaker:Amos Golan, American University
Time:Tuesday, June 11, 15:00-16:00
Place:Room 216, Guanghua Building 2
Abstract:
Our classical statistical arsenal for extracting truth from data often fails to produce correct predictions. Uncertainty, blurry evidence and multiple possible solutions may trip up even the best interrogator. Info-metrics – the science of modeling, reasoning, and drawing inferences under conditions of noisy and insufficient information – provides a consistent and efficient framework for constructing models and theories with minimal assumptions. It reveals the simplest solution, model or story, that is hidden in the observed information. Technically, info-metrics is at the intersection of information-theory and statistical inference. It combines the tools and principles of information theory, within a constrained optimization framework.
My talk will be based on my new book ‘Foundations of Info-Metrics: Modeling, Inference, and Imperfect Information,’ //info-metrics.org/ in which I develop and examine the theoretical underpinning of info-metrics and provide extensive interdisciplinary applications. In this talk I will discuss the basic ideas via a small number of graphical representations of the model and theory and will then present a number of interdisciplinary real-world examples for using the framework for inference. These examples include finance, network aggregation, predicting election, option pricing, and more. Depending on time, I will also discuss using that framework for basic modeling.
Introduction:
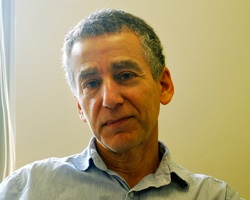
Amos Golan is a professor of economics and directs the Info-Metrics Institute at American University. He is also an External Professor at the Santa Fe Institute and a Senior Associate at Pembroke College, Oxford. His research is primarily in the interdisciplinary field of info-metrics - the science of modeling, reasoning, and drawing inferences under conditions of noisy and insufficient information. He has published in economics, econometrics, statistics, mathematics, physics, visualization and philosophy journals. His most recent book is ‘Foundations of Info-Metrics: Modeling, Inference, and Imperfect Information,’ Oxford University Press (2018). Web page://www.american.edu/cas/faculty/agolan.cfm
Your participation is warmly welcomed!