Statistics Seminar (2019-12)
Topic: An Asymptotic Theory of Least Squares Model Averaging
Speaker: Fang Fang, East China Normal University
Time: Friday, May 24, 14:00-15:00
Place: Room 217, Guanghua Building 2
Abstract:
Model averaging has attracted abundant attentions from researchers in the past decades as it becomes a powerful forecasting tool in areas such as econometrics, social sciences and medical studies. Theoretical results of model averaging methods mainly focus on asymptotical optimality of the selected model weights and large sample properties of the weights and the weighted parameter estimates. However, even with the most basic least squares model averaging, a full theoretical picture has not been obtained yet. In this talk, I will present some asymptotic results of least squares model averaging under two different scenarios: (1) All candidate models are wrong. (2) The true model is included in the candidate models. The results are connected to the asymptotical results of the model selection methods AIC and BIC. Some related issues will be further discussed.
Introduction:
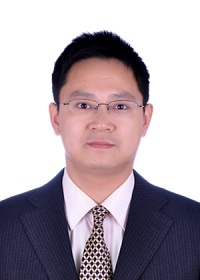
Fang Fang is Associate Professor of Key Laboratory of Advanced Theory and Application in Statistics and Data Science - MOE, School of Statistics, East China Normal University. He got his Bachelor degree at Peking University in 2002 and Ph.D. degree at University of Wisconsin - Madison in 2007. His research interests include model averaging, statistical learning and multisource data analysis.
Topic:Propensity model selection and Wilks phenomenon with nonignorable nonresponse
Speaker:Lei Wang, Nankai University
Time:Friday, May 24, 15:00-16:00
Place:Room 217, Guanghua Building 2
Abstract:
In this talk, two important issues caused by nonignorable nonresponse are investigated. We first propose a simultaneous propensity model and instrument selection criterion for identifiability and estimation. The proposed method can consistently select the most compact correct parametric propensity model and instrument from a group of candidate models, if one of these candidate models is correct and an instrument exists. We further show that Wilks's phenomenon does not hold due to the extra estimation of the nuisance parameter in the propensity, when applying the popular empirical likelihood to data with nonignorable nonresponses for constructing confidence regions. Motivated by this result, we propose an adjusted empirical likelihood for which Wilks's theorem holds. Asymptotic results and simulation results show that our proposed methods work quite well. Two real data examples are also presented for illustration.
Introduction:
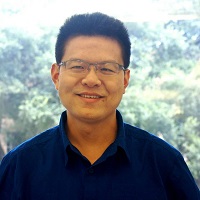
Lei Wang is currently Assistant Professor at School of Statistics and Data Science, Nankai University. He has been Postdoctoral Fellow at University of Wisconsin-Madison, and before that he obtains PhD(2014)degree from East China Normal University. His present research interests are in complex data analysis and empirical likelihood.
Your participation is warmly welcomed!