Statistics Seminar (2019-08)
Topic: Parsimonious Model Averaging with a Diverging Number of Parameters
Speaker: Xinyu Zhang,Academy of Mathematics and Systems Science, Chinese Academy of Sciences
Time: Thursday, Apr 25, 15:00-16:00
Place: Room 217, Guanghua Building 2
Abstract:
Model averaging generally provides better predictions than model selection, but the existing model averaging methods cannot lead to parsimonious models. Parsimony is an especially important property when the number of parameters is large. To achieve a parsimonious model averaging coefficient estimator, we suggest a novel criterion for choosing weights. Asymptotic properties are derived in two practical scenarios: (i) one or more correct models exist in the candidate model set; and (ii) all candidate models are misspecified. Under the former scenario, it is proved that our method can put the weight one to the smallest correct model and the resulting model averaging estimators of coefficients have many zeros and thus lead to a parsimonious model. The asymptotic distribution of the estimators is also provided. Under the latter scenario, prediction is mainly focused on and we prove that the proposed procedure is asymptotically optimal in the sense that its squared prediction loss and risk are asymptotically identical to those of the best – but infeasible – model averaging estimator. Numerical analysis shows the promise of the proposed procedure over existing model averaging and selection methods.
Introduction:
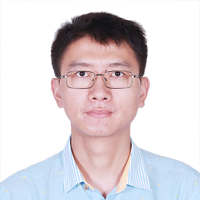
Xinyu Zhang, Postdoc, Texas A&M University, Ph.D., Chinese Academy of Sciences, Bachelor, Central University of Finance and Economics. RESEARCH INTERESTS: Model Selection and Averaging, Forecasting Combination, Estimation of Differential Equation Models.
Your participation is warmly welcomed!