Statistics Seminar (2019-06)
Topic: Estimation of Subgraph Densities in Noisy Networks
Speaker: Qiwei Yao, London School of Economics and Political Science
Time: Wednesday, Apr 10, 14:00-15:00
Place: Room 216, Guanghua Building 2
Abstract:
While it is common practice in applied network analysis to report various standard network summary statistics, these numbers are rarely accompanied by some quantification of uncertainty. Yet any error inherent in the measurements underlying the construction of the network, or in the network construction procedure itself, necessarily must propagate to any summary statistics reported. Here we study the problem of estimating the density of an arbitrary subgraph, given a noisy version of some underlying network as data. Under a simple model of network error, we show that consistent estimation of such densities is impossible when the rates of error are unknown and only a single network is observed. Next, focusing first on the problem of estimating the density of edges from noisy networks, as a canonical prototype of the more general problem, we develop method-of-moment estimators of network edge density and error rates for the case where a minimal number of network replicates are available. These estimators are shown to be asymptotically normal as the number of vertices increases to infinity. We also provide confidence intervals for quantifying the uncertainty in these estimates based on either the asymptotic normality or a bootstrap scheme. We then present a generalization of these results to higher-order subgraph densities, and illustrate with the case of two-star and triangle densities. Bootstrap confidence intervals for those high-order densities are constructed based on a new algorithm for generating a graph with pre-determined counts for edges, two-stars, and triangles. The algorithm is based on the idea of edge-rewiring, and is of some independent interest. We illustrate the use of the proposed methods in the context of gene coexpression networks.
Introduction:
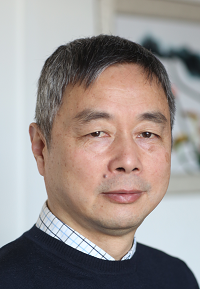
Qiwei’s recent research has mainly (but not exclusively) focused on analysing complex and high-dimensional time series in the sense that the observation recorded at each time is more complex than a single scalar or a short vector. Two examples under this framework are spatio-temporal data and dynamic network for which the observation at each time is a space or a network. Demand for analysing those data on an ever-increasing scale is a part of the real challenge underneath the buzzword BigData. The time series thinking and methodology can contribute in facing those challenges. An overarching aim has been to develop new tools which reduce the dimension and/or the complexity of time series by exploring latent low dimensional structures.
Your participation is warmly welcomed!